Advancements in Federating Learning Highlighted in Papers Presented at ICCV 2023
PPML
October 6, 2023
As the field of machine learning continues to evolve, Sony AI researchers are constantly exploring innovative solutions to address the pressing issues faced by the industry. Two research papers, both accepted at the premier international computer vision event ICCV 2023 , shed light on significant challenges in the realm of federated learning (FL) and propose groundbreaking methods to overcome them. In this blog, we'll explore these papers and their potential implications.
The first paper, Addressing Catastrophic Forgetting in Federated Class-Continual Learning, delves into a relatively unexplored but crucial problem: how to adapt to the dynamic emergence of new concepts in a federated setting. The second paper, MAS: Towards Resource-Efficient Federated Multiple-Task Learning, addresses the challenge that arises when multiple FL tasks need to be handled simultaneously on resource-constrained devices.
Both of these papers address critical difficulties in the domain of FL, an emerging distributed machine learning method that empowers in-situ model training on decentralized edge devices. FL has gained significant attention for its ability to enable privacy-preserving distributed model training among decentralized devices. However, it comes with its own set of obstacles , and these papers tackle two of the most pressing ones.
TARGET: Addressing Catastrophic Forgetting in Federated Class-Continual Learning
Consider the scenario of multiple health institutions collaborating using FL to identify new strains of a virus like COVID-19. As new strains continually emerge due to the virus's high mutation rate, the need for a robust and efficient learning approach becomes apparent. This highlights a challenge in FL to balance new knowledge gained from updates while preserving the knowledge the model has already acquired so that the model can be continuously improved without losing its previous abilities.
Traditionally, one might think of training new models from scratch to accommodate these emerging classes. However, this approach is computationally intensive and impractical. Alternatively, one could turn to transfer learning from previously trained models, but it often leads to catastrophic forgetting, where the model's performance in previous classes deteriorates significantly.
To address these challenges, recent research introduced the concept of Continual Learning (CL) within the FL framework, collectively known as Federated Continual Learning (FCL). Figure 1. illustrates the setting of FCL, where data in each FL client is continuously changing over time.
FCL methods aim to mitigate catastrophic forgetting in FL. One particular scenario where new classes are continually added is termed Federated Class-Continual Learning (FCCL).
This paper is the first work to highlight that non-independent and Identically Distributed (non-IID) data worsens the catastrophic forgetting issue in FL. The proposed solution, TARGET (Federated clAss-continual leaRninG via Exemplar-free disTillation), is a new method for FCCL. It leverages the previously trained global model to transfer knowledge from old classes to the current one at the model level. This ensures that the model retains its proficiency in previous classes while learning new ones. In addition, TARGET trains a generator to produce synthetic data that mimics the global data distribution on each client at the data level.
Figure 1 illustrates the pipeline of TARGET that leverages both the trained global model and synthetic data to mitigate the imbalanced distribution of data among clients, a key factor exacerbating catastrophic forgetting.
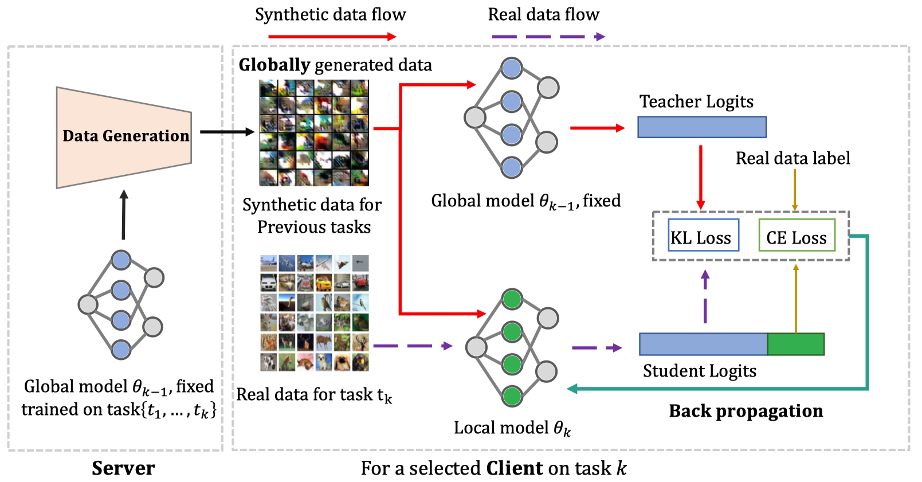
Figure1. Pipeline of our proposed TARGET.
The potential applications of TARGET are vast. For example, in healthcare, where data privacy is paramount, TARGET can facilitate continuous model training for disease detection while respecting strict data regulations. In autonomous vehicles, it can adapt to new traffic conditions and scenarios without sacrificing performance on previously learned tasks.
MAS(Merge and Split): Towards Resource-Efficient Federated Multiple-Task Learning
FL has garnered significant attention for its ability to enable privacy-preserving distributed model training among decentralized devices. However, the challenge arises when multiple FL tasks need to be handled simultaneously on resource-constrained devices.
In fact, most edge devices can typically support only one FL task at a time. Introducing multiple simultaneous FL tasks on the same device can lead to memory overload, excessive computation, and power consumption. This bottleneck presents a significant challenge in scenarios where multiple tasks need to be executed simultaneously.
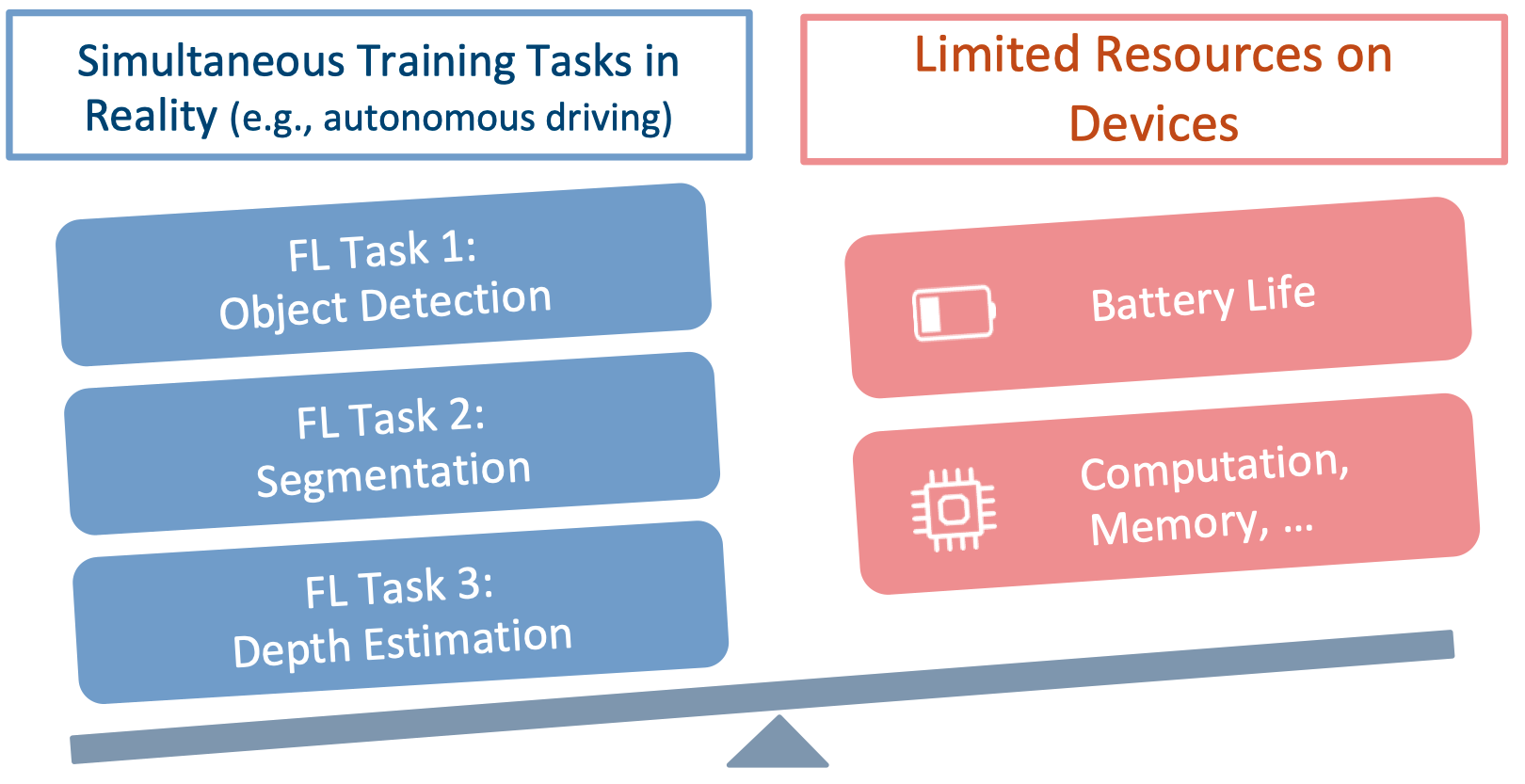
Figure 2. The tussle of training simultaneous FL tasks on resource-constrained devices.
Existing approaches have their limitations. Some train tasks sequentially, leading to slow convergence, while others employ multi-task learning but may overlook the unique requirements of each task.
This paper is the first work that conducts an in-depth investigation into the training of simultaneous FL tasks. Our proposed method, MAS (Merge and Split), presents a novel solution to this problem. It balances the synergies and differences among multiple simultaneous FL tasks. Figure 3 depicts the architecture and workflow of MAS. It starts by merging these FL tasks into an all-in-one FL task with a multi-task architecture. After training the all-in-one task for a certain number of rounds, MAS employs a clever splitting mechanism, considering the affinities among tasks. It then continues training each split of FL tasks with models trained in the all-in-one process. MAS achieves the best performance while reducing training time by 2x and energy consumption by 40%.
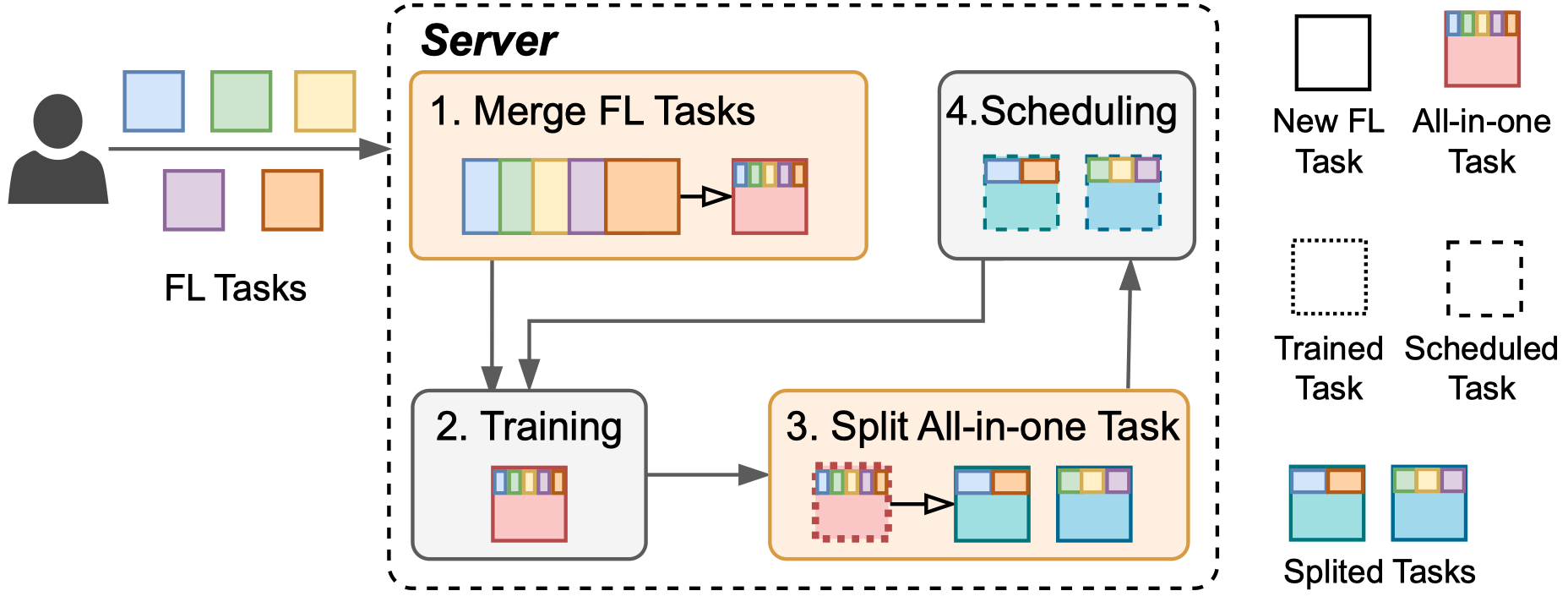
Figure 3. MAS (Merge and Split) architecture and workflow.
MAS's potential applications are profound. In fields like autonomous vehicles, robotics, and intelligent manufacturing, where multiple resource-intensive tasks need to be executed simultaneously, MAS can optimize training, reduce energy consumption, and improve overall model performance. It streamlines the process of training multiple tasks on a single device, opening doors to more efficient and effective implementations of FL.
In conclusion, these two papers accepted at ICCV 2023 highlight the continuous innovation in the field of FL. TARGET and MAS address critical challenges, paving the way for more efficient, privacy-aware, and adaptable machine learning models. As researchers and practitioners continue to explore the possibilities, we can anticipate these technologies making a significant impact in various industries, from healthcare to autonomous vehicles, and beyond.
Latest Blog
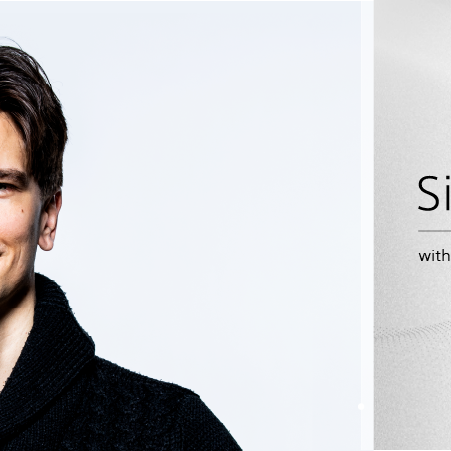
June 18, 2024 | Sony AI
Sights on AI: Tarek Besold Offers Perspective on AI for Scientific Discovery, Ba…
The Sony AI team is a diverse group of individuals working to accomplish one common goal: accelerate the fundamental research and development of AI and enhance human imagination an…
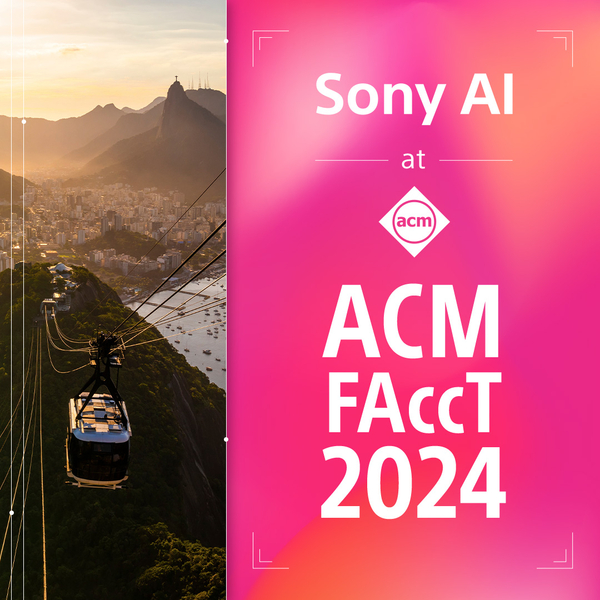
June 4, 2024 | Events , Sony AI
Not My Voice! A Framework for Identifying the Ethical and Safety Harms of Speech…
In recent years, the rise of AI-driven speech generation has led to both remarkable advancements and significant ethical concerns. Speech generation can be a driver for accessibili…
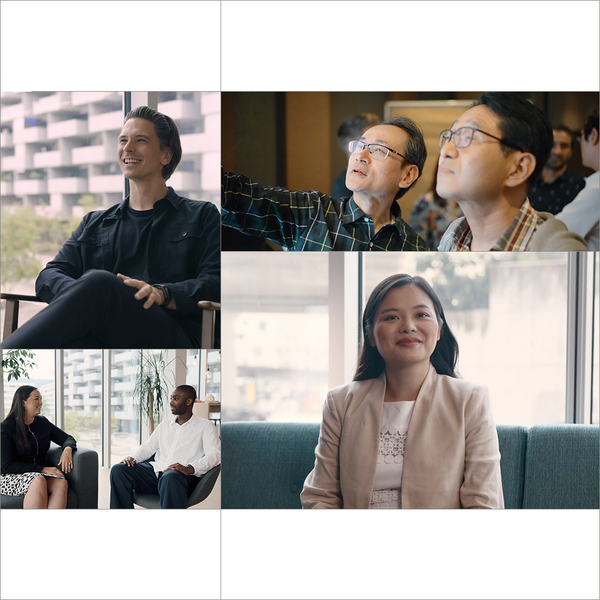
May 22, 2024 | Sony AI
In Their Own Words: Sony AI’s Researchers Explain What Grand Challenges They’re …
This year marks four years since the inception of Sony AI. In light of this milestone, we have found ourselves reflecting on our journey and sharpening our founding commitment to c…