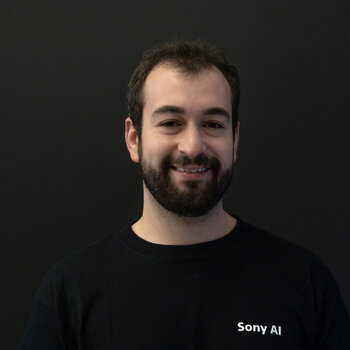
Roberto
Capobianco
Profile
Roberto is a research scientist at Sony AI and external professor at Sapienza University of Rome. Previously, he was a research scientist at Cogitai and an assistant professor at Sapienza University of Rome, where he also received his Ph.D., under the supervision of Prof. Daniele Nardi. Roberto was a visiting Research Scholar at the Robotics Institute - Carnegie Mellon University (Pittsburgh, USA), under the supervision of Prof. J. Andrew Bagnell. He has been awarded a 3-years fellowship, a Research Starting Grant by Sapienza University of Rome, and a Robotics Fellowship in 2016 by AAAI. Roberto completed his MSc in AI and robotics in 2013, at Sapienza University of Rome, and his BSc in computer engineering at University of Pisa in 2011.
Message
“At Sony AI, I currently focus my research on reinforcement learning, including model-based approaches, and robot learning. I am also very interested in Explainable AI and Ethics. My long-term goal at Sony is to create ethical learning agents that can interact and communicate with humans in dynamic environments/domains, to empower their creativity and self-realization capabilities. At the same time, I really hope that through such empowered creativity we can better understand what intelligence really is.”
Publications
Compositional Explanations is a method for identifying logical formulas of concepts that approximate the neurons' behavior. However, these explanations are linked to the small spectrum of neuron activations used to check the alignment (i.e., the highest ones), thus lacking c…
Two of the most impressive features of biological neural networks are their high energy efficiency and their ability to continuously adapt to varying inputs. On the contrary, the amount of power required to train top-performing deep learning models rises as they become more …
Molecular property prediction is a fundamental task in the field of drug discovery. Several works use graph neural networks to leverage molecular graph representations. Although they have been successfully applied in a variety of applications, their decision process is not t…
Deep reinforcement learning (DRL) models have shown great promise in various applications, but their practical adoption in critical domains is limited due to their opaque decision-making processes. To address this challenge, explainable AI (XAI) techniques aim to enhance tra…
A critical challenge in neuro-symbolic (NeSy) approaches is to handle the symbol grounding problem without direct supervision. That is mapping high-dimensional raw data into an interpretation over a finite set of abstract concepts with a known meaning, without using labels. …
Non-markovian Reinforcement Learning (RL) tasks are extremely hard to solve, because intelligent agents must consider the entire history of state-action pairs to act rationally in the environment. Most works use Linear Temporal Logic (LTL) to specify temporally-extended task…
Deep reinforcement learning has achieved impressive results in recent years; yet, it is still severely troubled by environments showcasing sparse rewards. On top of that, not all sparse-reward environments are created equal, ie, they can differ in the presence or absence of …
Deep neural networks are the driving force of the recent explosion of machine learning applications in everyday life. However, they usually require a lot of training data to work well, and they act as black-boxes, making predictions without any explanation about them. This p…
The use and creation of machine-learning-based solutions to solve problems or reduce their computational costs are becoming increasingly widespread in many domains. Deep Learning plays a large part in this growth. However, it has drawbacks such as a lack of explainability an…
Graph neural networks have proved to be a key tool for dealing with many problems and domains such as chemistry, natural language processing and social networks. While the structure of the layers is simple, it is difficult to identify the patterns learned by the graph neural…
A critical challenge in neurosymbolic approaches is to handle the symbol grounding problem without direct supervision. That is mapping high-dimensional raw data into an interpretation over a finite set of abstract concepts with a known meaning, without using labels. In this …
The main protease (Mpro) of SARS-Cov-2 is the essential enzyme for maturation of functional proteins implicated in viral replication and transcription. The peculiarity of its specific cleavage site joint with its high degree of conservation among all coronaviruses promote it…
This work aims to present a method to perform autonomous precision landing—pin-point landing—on a planetary environment and perform trajectory recalculation for fault recovery where necessary. In order to achieve this, we choose to implement a Deep Reinforcement Learning—DRL…
Many potential applications of artificial intelligence involve making real-time decisions in physical systems while interacting with humans. Automobile racing represents an extreme example of these conditions; drivers must execute complex tactical manoeuvres to pass or block…
Planetary rovers have a limited sensory horizon and operate in environments where limited information about the surrounding terrain is available. The rough and unknown nature of the terrain in planetary environments potentially leads to scenarios where the rover gets stuckan…
NeuroEvolution Strategies (NES) are a subclass of Evolution Strategies (ES). While their application to games and board games have been studied in the past [11], current state of the art in most of the games is still held by classic RL models, such as AlphaGo Zero [16]. This…
Sparse-reward environments are famously challenging for deep reinforcement learning (DRL) algorithms. Yet, the prospect of solving intrinsically sparse tasks in an end-to-end fashion without any extra reward engineering is highly appealing. Such aspiration has recently led t…
The recent success of deep learning models in solving complex problems and in different domains has increased interest in understanding what they learn. Therefore, different approaches have been employed to explain these models, one of which uses human-understandable concept…
The recent explosion of deep learning techniques boosted the application of Artificial Intelligence in a variety of domains, thanks to their high performance. However, performance comes at the cost of interpretability: deep models contain hundred of nested non-linear operati…
In this work, we develop an application for autonomous landing, exploiting the properties of Deep Reinforcement Learning and Transfer Learning in order to tackle the problem of planetary landing on unknown or barely-known extra-terrestrial environments by learning good-perfo…
The year 2020 saw the covid-19 virus lead to one of the worst global pandemics in history. As a result, governments around the world have been faced with the challenge of protecting public health while keeping the economy running to the greatest extent possible. Epidemiologi…
The aim of this work is to develop an application for autonomous landing. We exploit the properties of Deep Reinforcement Learning and Transfer Learning, in order to tackle the problem of planetary landing on unknown or barely-known extra-terrestrial environments by learning…
This paper addresses an epidemiologic inference problem where, given realtime observation of test results, presence of symptoms,and physical contacts, the most likely infected individuals need to be inferred. The inference problem is modeled as a hidden Markovmodel where inf…
In this paper we present a novel mechanism to get explanations that allow to better understand network predictions when dealing with sequential data. Specifically, we adopt memory-based networks — Differential Neural Computers — to exploit their capability of storing data in…
The year 2020 has seen the COVID-19 virus lead to one of the worst global pandemics in history. As a result, governments around the world are faced with the challenge of protecting public health, while keeping the economy running to the greatest extent possible. Epidemiologi…
Blog
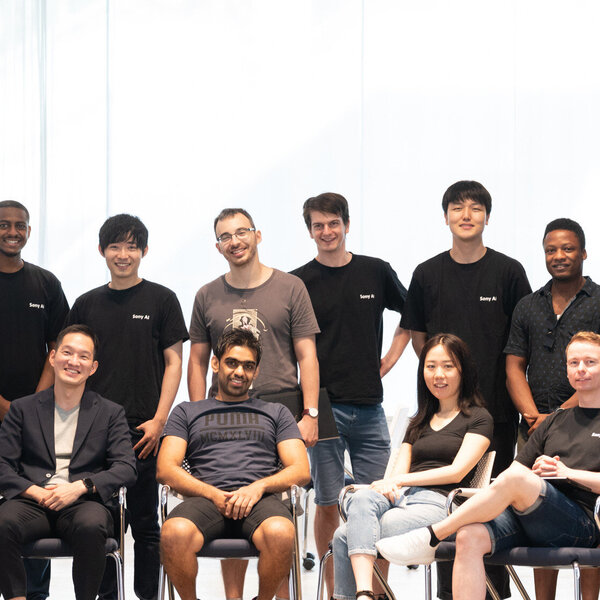
November 29, 2021 | Life at Sony AI
Meet the Team #2: Lingjuan, Jerone and Roberto
What do privacy, pattern recognition, and percussion all have in common? They are concepts and creative endeavors that have inspired Sony AI team members Lingjuan, Jerone and Roberto. Read on to learn more about these three Sony…
What do privacy, pattern recognition, and percussion all have in common? They are concepts and creative endeavors that have insp…
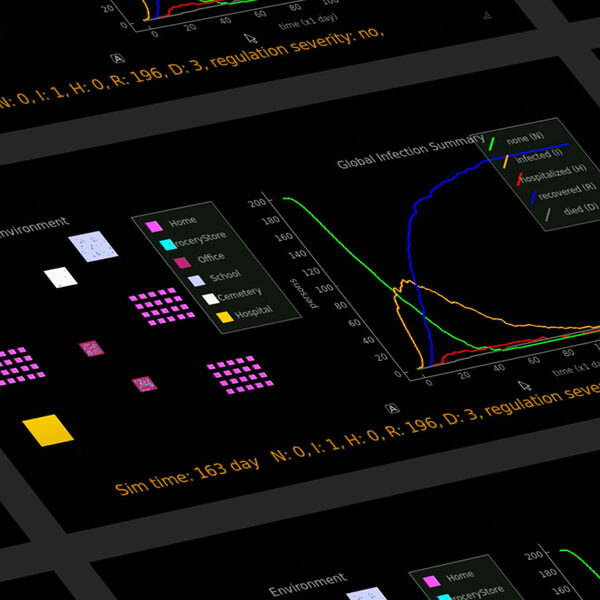
March 3, 2021 | Life at Sony AI
The Challenge to Create a Pandemic Simulator
The thing I like most about working at Sony AI is the quality of the projects we're working on, both for their scientific challenges and for their potential for improving the world. What could be more exciting than magnifying hu…
The thing I like most about working at Sony AI is the quality of the projects we're working on, both for their scientific challen…
JOIN US
Shape the Future of AI with Sony AI
We want to hear from those of you who have a strong desire
to shape the future of AI.